PhD Defense - Ahmed Shama (WP8 SFC) - Data-Driven Predictive Models: Calculational Bias in Characterization of Spent Nuclear Fuel
We are pleased to announce the public defense for the PhD Dissertation of Ahmed Shama. The title of Ahmed Shama’s PhD thesis is “ Data-Driven Predictive Models: Calculational Bias in Characterization of Spent Nuclear Fuel ”. Ahmed Shama performed his PhD in the frame of EURAD WP8 Task 2.
January 31st, 2022
10-00-12.00
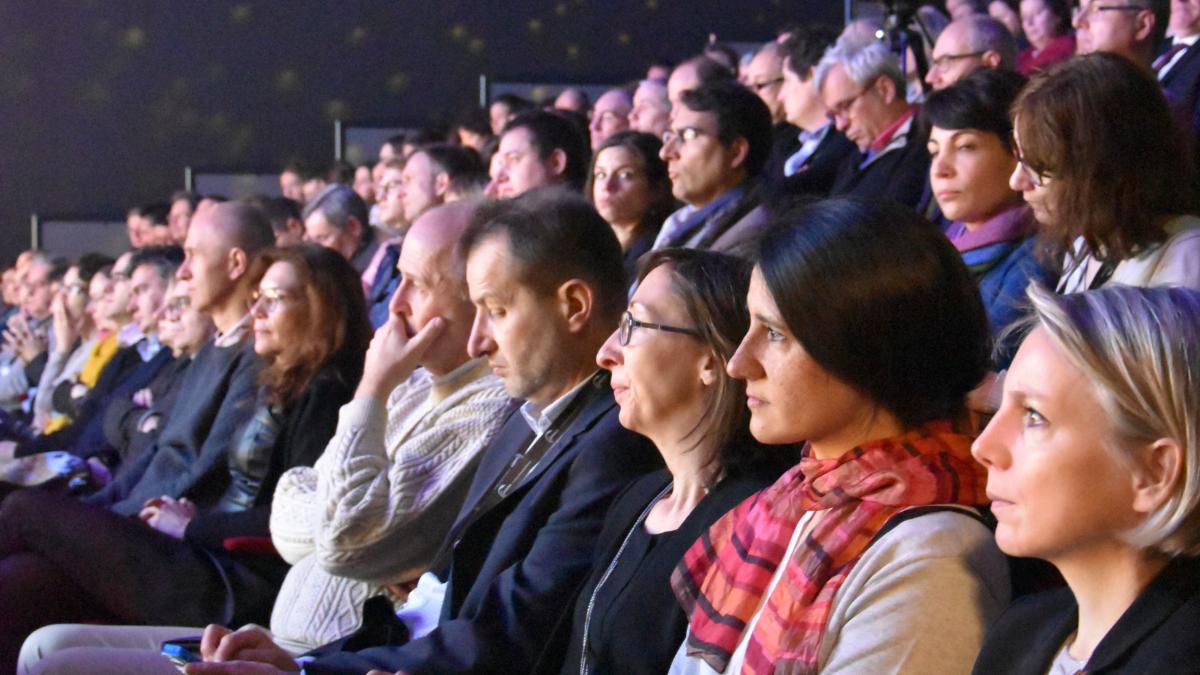
Thesis director: Prof. Dr. Andreas Pautz
Thesis co-director: Dr. Dimitri Rochman
Abstract
Characteristics of the spent nuclear fuel (SNF) are typically calculated, requiring validation a priori. The validation process relies on the difference between calculations and measurements, namely the bias. Usually, predicting the bias based on benchmarks is essential, which motivated the present research, focusing on SNF decay heat and Cs-137, U-235, and Pu-239 concentrations. The validation benchmarks are from open-literature, i.e., SNF design and irradiation specifications, as well as the measurements of their characteristics. For the decay heat, they correspond to 262 measurements, conducted at the Clab and the GE-Morris facilities. For the radionuclide concentrations, they are 285 post-irradiation-examination samples, obtained from the SFCOMPO database. The calculations rely on the SCALE code system, namely the Polaris code and the SCALE-based nuclear data.
Uncertainties of nuclear data and SNF design and operational history are propagated to the calculated quantities to obtain correlation matrices between the benchmarks. Then, machine learning (ML) methods are applied to predict the bias of calculated SNF characteristics. The predictive performance is analyzed by comparing the ML-based bias predictions and the validation-based biases. The analyzed ML models predict the bias using highly similar benchmarks or neighbors of the benchmarks, namely Random Forests (RF) and Weighted k-Nearest Neighbors (KKNN). Also, the linear model is analyzed.
This research shows that the bias of the decay heat and Pu-239 concentration can be predicted with a reasonable accuracy, relying on specific features of validation benchmarks, or their correlations. The predicted biases bear statistically significant similarities to the observed ones from the validation procedure, using both the RF and the KKNN models. The variances in the original validation data are significantly reduced. The models predict the bias using the spectral index for the decay heat and the hydrogen-to-fissile atom ratio for the Pu-239 concentration. Also, the correlation matrices show that they are informative in predicting the bias of both characteristics. In the case of the U-235 and Cs-137 concentrations, biases could not be satisfactorily predicted. Additionally, the linear models have shown unsatisfactory performance.